Understanding RAG
When generative AI meets information retrieval for more relevant and accurate answers
In a world where artificial intelligence (AI) technologies are evolving at breakneck speed, Retrieval Augmented Generation (RAG) stands out as a revolutionary approach. Combining text generation and the search for relevant information, RAG opens up new perspectives for AI-based applications. In this article, Gaël Yvrard, Project Director of our Search and Semantic entity, and Jean-louis Vila, CTO of Coexya, present the added value provided by RAG.
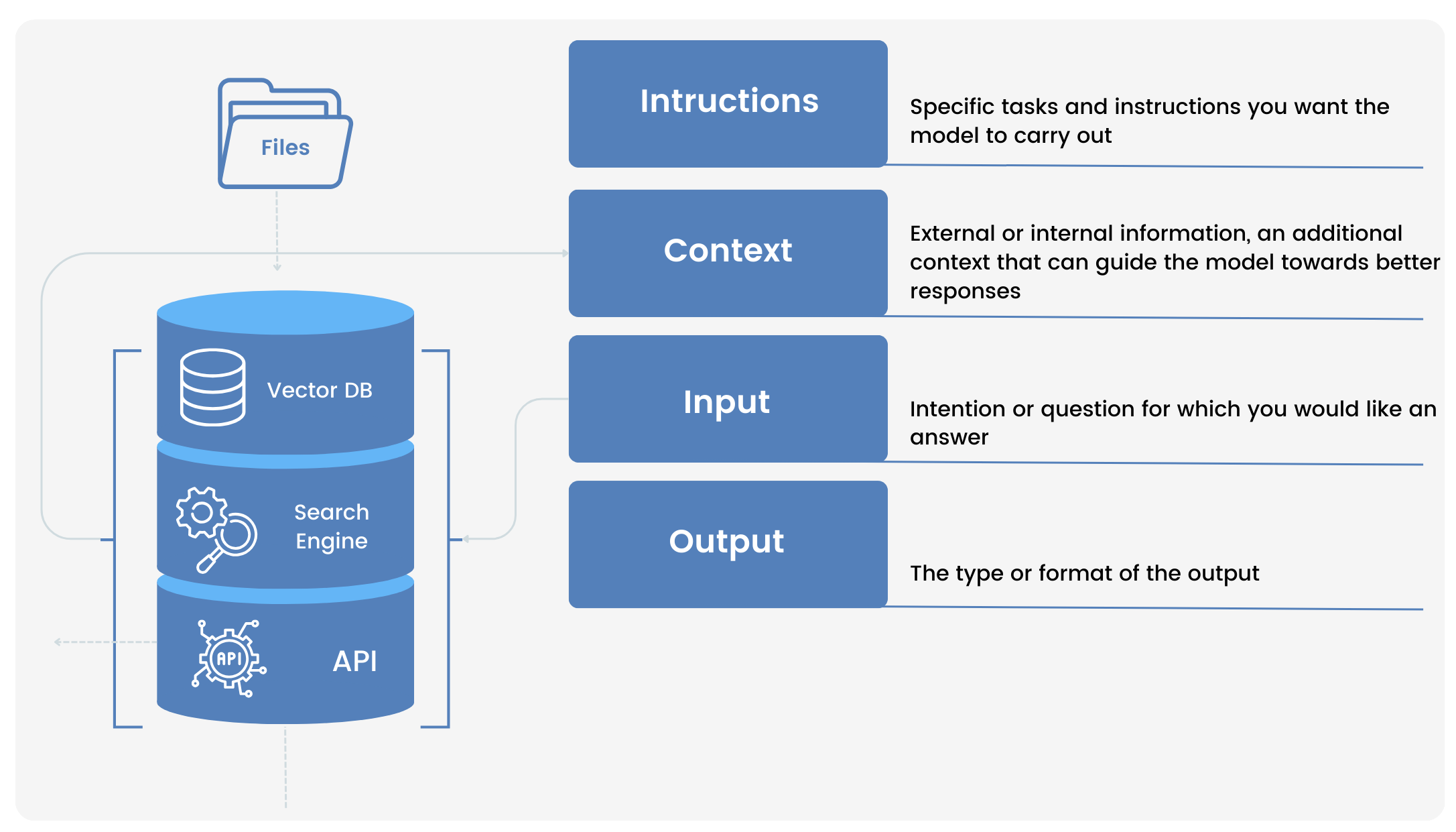
What is RAG and why is it necessary in generative AI?
For generative AI, either the knowledge is contained in the model itself, or it is provided by a third-party component. The original idea behind RAG is to automatically inject relevant elements into the context in order to generate a more accurate response.
This approach consists of searching for information in different data sources based on the user’s intentions.
A classic RAG approach is the projection (embeddings) of information onto a multi-dimensional space, the output of which is a vector. The user’s intentions/questions are themselves projected into the same space, which makes it possible to calculate a similarity or distance with the vectorised information and thus return the most relevant information.
This data is then injected into the context that will be used to generate the response by the generative AI.
RAG: What are the main use cases?
RAG is used in many areas to improve access and the accuracy of the information returned:
- Document search: In areas where there are large numbers of documents, the RAG enables a precise and synthetic search. As well as returning the relevant documents, it produces a summary of all the documents.
- Customer support: RAG-enabled chatbots can search manuals or user guides to answer customers’ questions accurately;
- Interaction with documents: enables documents to be queried from different angles: summary, translation, comparisons, questions, etc.
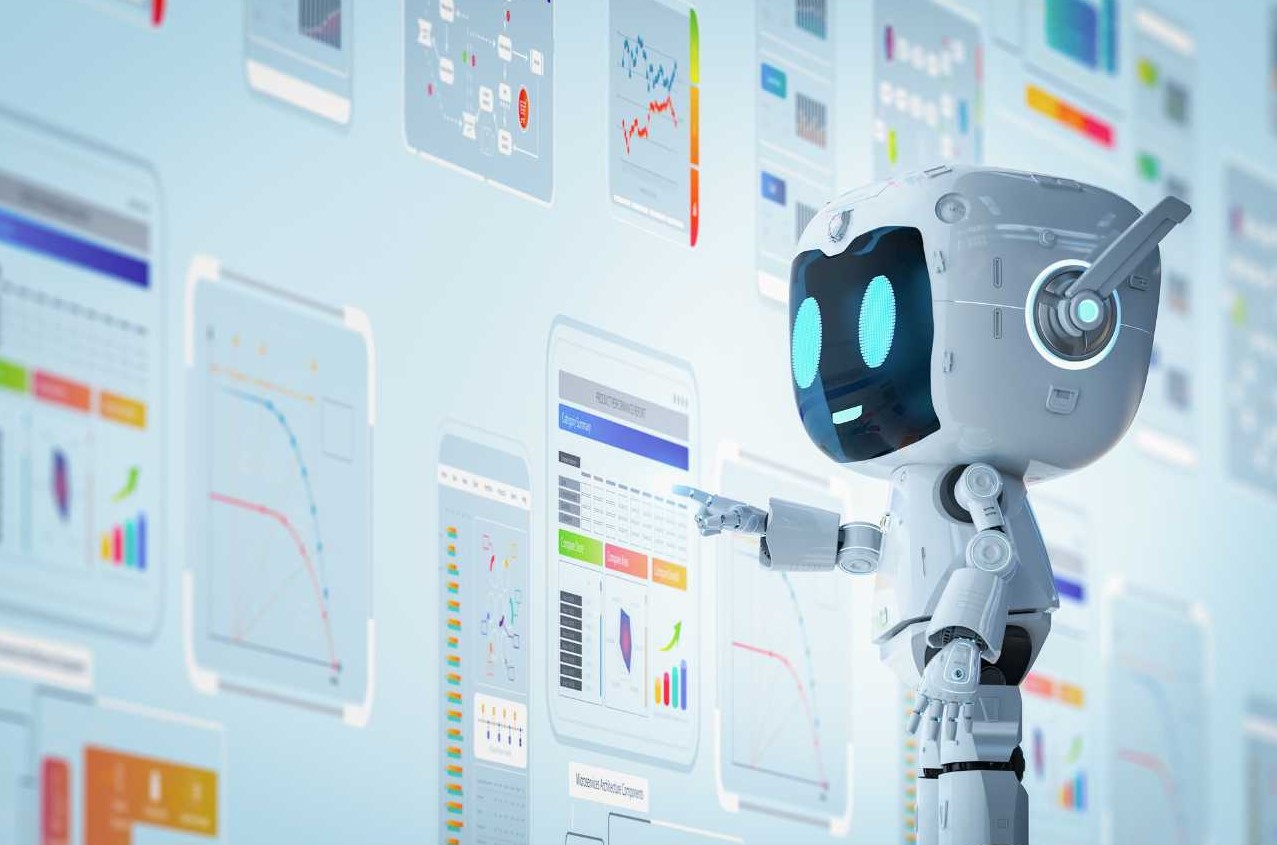
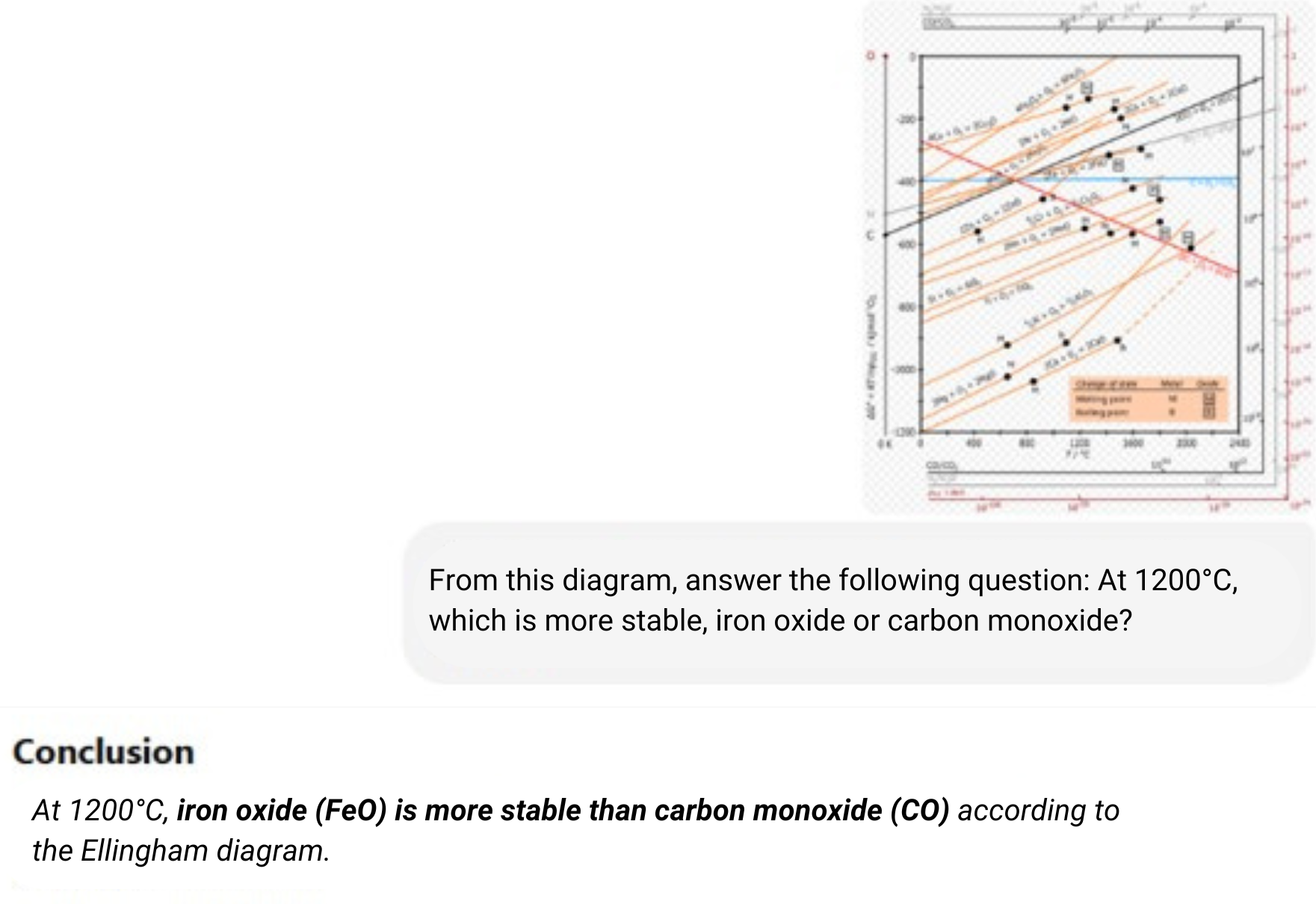
The benefits of RAG: Why adopt it?
- Increased accuracy: Unlike LLMs, RAG increases accuracy and confidence by relying on sourced and verifiable information;
- Adaptability: solutions implementing RAG can index different knowledge bases, even when the data is unstructured, making them particularly versatile;
- Improved user experience: The answers provided by the RAG are personalised according to the user’s context;
- Reduced costs: By simplifying and accelerating document searches, the RAG saves users valuable time, reducing the costs associated with manual information retrieval.
The future of RAG
Until now, RAG has worked by arbitrarily slicing up documents to associate passages relevant to the user’s question, before proposing a summary. While this approach has the advantage of being simple and quick to implement, it also has a number of limitations:
- Limited access to non-textual data: information contained in graphs, images or tables cannot be used.
- Lack of global vision: the answer must be found directly in a paragraph of the documents used, preventing a broader analysis.
- Lack of reasoning and adaptability: the model cannot interpret a complex context or draw implicit conclusions.
Faced with these limitations, new approaches are emerging:
- LMMs (Large Multimodal Models) can be used to analyse a wide range of content, including images and tables.
- GraphRAG uses a knowledge graph to exploit implicit information. For example, if Mr Dupont has written a document on a technology, we can deduce that he has mastered it.
- Agentic RAG is based on agents capable of reasoning, planning and interacting proactively with knowledge bases.
RAG, a strategic lever for the future of AI
The RAG is an ingenious development in the field of AI that makes it possible to further improve the power of generative AI by combining search and text generation.